In today’s fast-paced financial markets, traders and investors rely on financial signals to make informed decisions. These signals, derived from various sources such as price movements, trading volume, economic indicators, and even social sentiment, help identify potential market trends and opportunities. However, extracting meaningful insights from these signals can be challenging due to market noise, volatility, and ever-changing economic conditions.
This is where financial signal processing and machine learning come into play. Traditional trading strategies often rely on technical and fundamental analysis, but AI-driven models revolutionize how economic data is analyzed and used. Machine learning algorithms can process vast amounts of data, detect patterns, and make real-time trading decisions faster and more accurately than humans.
From algorithmic trading and sentiment analysis to fraud detection and risk management, integrating financial signal processing with machine learning is reshaping the economic landscape. This technology enhances decision-making and helps traders mitigate risks and maximize profitability.
In this article, we will explore the concept of financial signals, how they are processed, and the role of machine learning in revolutionizing trading strategies.
What is a Financial Signal?
A financial signal is a data-driven indicator that helps traders and investors make buy, sell, or hold decisions in financial markets. These signals can be generated from various sources, including price movements, market trends, economic data, news sentiment, and technical indicators.
Financial signals can be broadly classified into two main types:
- Technical Signals – Derived from price action and trading volume, these signals help traders identify market trends and momentum. Common technical indicators include:
- Moving Averages (MA) – Helps smooth out price fluctuations to identify trends.
- Relative Strength Index (RSI) – Measures momentum to detect overbought or oversold conditions.
- MACD (Moving Average Convergence Divergence) – A trend-following indicator that signals bullish or bearish momentum.
- Fundamental Signals – Based on macroeconomic factors and financial statements, these signals help investors assess a company’s or market’s intrinsic value. Examples include:
- Earnings Reports – Strong earnings growth can signal a good investment opportunity.
- Interest Rates and Inflation – Changes in central bank policies impact market sentiment.
- Market Sentiment Analysis – AI-driven sentiment analysis scans news, social media, and financial reports to detect shifts in investor mood.
While traditional traders manually interpret financial signals, financial signal processing and machine learning have automated and enhanced this process. By leveraging AI, traders can filter out market noise, identify high-probability trading opportunities, and make data-driven investment decisions more accurately.
Next, we’ll dive deeper into how financial signal processing works and how machine learning improves its effectiveness in trading.
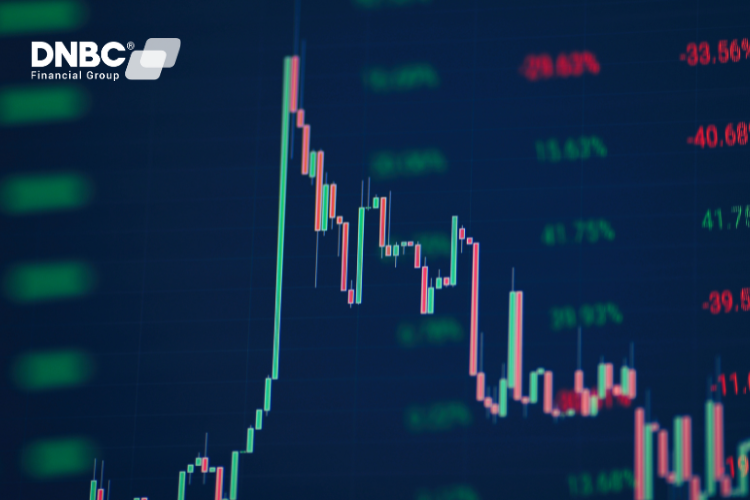
The Role of Financial Signal Processing in Trading
Financial markets generate vast amounts of data every second, making it difficult for traders to extract valuable insights amid the noise. Financial signal processing is crucial in filtering, analyzing, and interpreting this data to identify reliable trading signals.
At its core, financial signal processing applies mathematical and statistical techniques to detect patterns, remove irrelevant data, and enhance decision-making. Some of the key methods used in financial signal processing include:
- Fourier Transform – This breaks down complex market data into simpler frequency components, helping traders detect cyclic trends.
- Wavelet Transform – Allows traders to analyze financial data at different time scales, identifying short-term and long-term trends.
- Kalman Filters – Used to smooth and predict financial time series, improving the accuracy of forecasting models.
- Hidden Markov Models (HMM) – Helps identify market regime changes by recognizing shifts in price behavior.
Financial institutions, hedge funds, and high-frequency traders use these advanced signal-processing techniques to improve trend detection, volatility analysis, and risk assessment. By refining raw market data into actionable insights, financial signal processing minimizes false signals and improves trading accuracy.
However, traditional signal processing has its limitations. While it enhances data interpretation, it relies on human-designed models, which may not constantly adapt well to rapid market fluctuations. This is where machine learning takes financial signal processing to the next level, automating complex data analysis and improving predictive accuracy in trading.
Next, we explore how machine learning revolutionizes financial signal processing, leading to more innovative, AI-driven trading strategies.
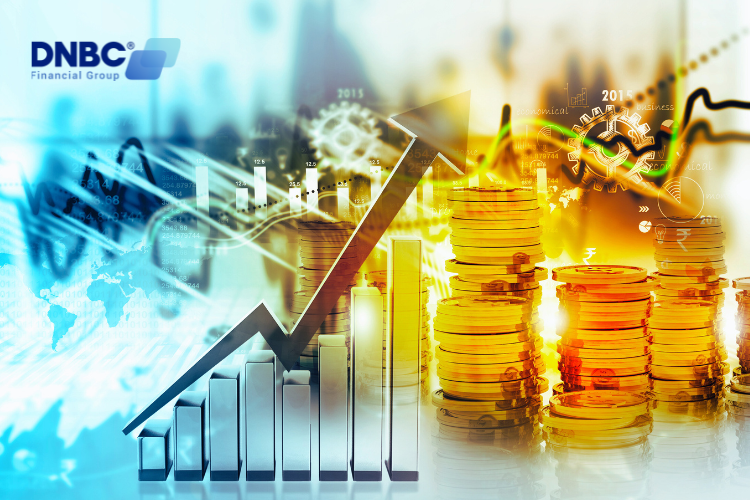
How Machine Learning Enhances Financial Signal Processing
While traditional financial signal processing methods are effective, they often struggle with dynamic and unpredictable market conditions. Machine learning (ML) bridges this gap by allowing models to adapt, learn from new data, and improve predictions over time.
Here’s how machine learning enhances financial signal processing:
1. Pattern Recognition & Trend Prediction
Machine learning algorithms can detect complex, non-linear relationships in financial signals that traditional models might miss. Key techniques include:
- Supervised Learning – Uses labeled historical data to train models that predict future price movements (e.g., decision trees, random forests).
- Deep Learning & Neural Networks – Advanced ML techniques that recognize subtle price movement patterns, leading to more accurate trading signals.
2. Anomaly Detection & Risk Management
Financial markets are prone to shocks, manipulation, and anomalies that can disrupt trading strategies. ML-powered anomaly detection:
- Identifies market inefficiencies that can be exploited for profit.
- Detects potential fraud or unusual trading patterns using unsupervised learning techniques.
3. Real-Time Trading & High-Frequency Trading (HFT)
Machine learning enables automated, real-time analysis of financial signals, allowing hedge funds and institutional traders to execute trades within milliseconds.
- Reinforcement Learning helps AI-driven trading bots learn from past decisions and refine strategies dynamically.
- Sentiment Analysis with NLP (Natural Language Processing) processes news, financial reports, and social media to assess market sentiment as a trading signal.
By integrating financial signal processing and machine learning, modern traders gain a competitive advantage with data-driven, adaptive, and precise trading strategies. AI-driven models reduce human bias, minimize errors, and improve profitability by responding to market signals in real-time.
Next, we’ll explore real-world machine learning applications in financial trading, from algorithmic trading to risk management.
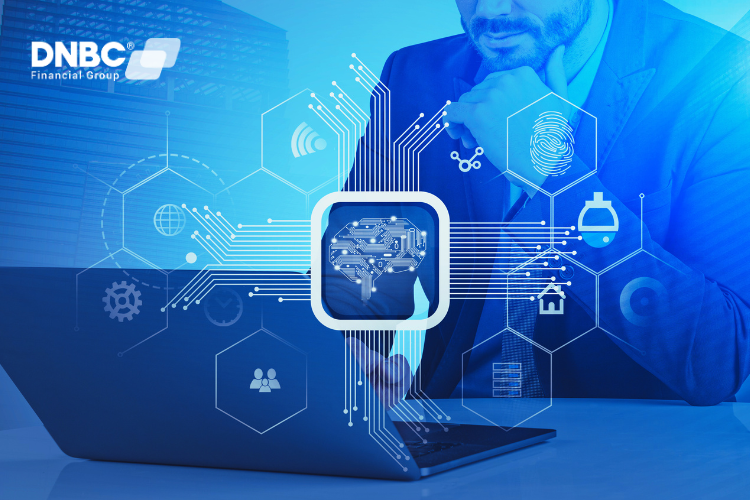
Real-World Applications: How Traders Use Financial Signal Processing and Machine Learning
The integration of financial signal processing and machine learning has led to groundbreaking advancements in trading. These technologies are reshaping how financial markets operate, from algorithmic trading to fraud detection. Below are some of the most impactful applications:
1. Algorithmic Trading & High-Frequency Trading (HFT)
- Machine learning models analyze historical and real-time financial signals to automate trade execution.
- High-frequency trading (HFT) firms use AI-driven strategies to execute millisecond trades, capitalizing on short-term price fluctuations.
- Example: Renaissance Technologies, a hedge fund, uses machine learning models to predict price movements based on financial signals.
2. Sentiment Analysis in Trading
- Natural Language Processing (NLP) scans financial news, analyst reports, and social media to gauge market sentiment.
- Example: AI-driven sentiment analysis detected negative investor sentiment before the GameStop stock crash, helping traders adjust their positions.
3. Risk Management & Portfolio Optimization
- Machine learning models assess risk exposure by analyzing volatility, economic indicators, and trading patterns.
- AI-powered risk management systems predict market downturns and adjust portfolios automatically.
4. Fraud Detection & Insider Trading Prevention
- Financial institutions use ML algorithms to detect unusual trading patterns that may indicate fraud.
- AI flags transactions that deviate from normal trading behaviors helping regulators catch market manipulation schemes.
With these applications, financial institutions, hedge funds, and retail traders leverage financial signal processing and machine learning to improve accuracy, reduce risk, and maximize profitability. However, despite these advancements, challenges remain. The following section will explore the limitations and risks associated with AI-driven financial trading.
Conclusion
Integrating financial signal processing and machine learning revolutionizes trading, enabling investors and businesses to make smarter, faster, and more data-driven financial decisions. From algorithmic trading and sentiment analysis to risk management and fraud detection, AI-powered financial tools transform markets’ operations. However, while these technologies bring numerous advantages, data quality, market unpredictability, and ethical considerations remain essential challenges.
At DNBC Financial Group, we recognize the power of AI-driven financial solutions and the importance of personal service in navigating today’s complex economic landscape. Whether you’re managing international transfers, optimizing investments, or streamlining business services, our commitment is to provide you with secure, efficient, and intelligent financial solutions. As technology continues to evolve, DNBC remains at the forefront, combining innovation with personalized support to meet the unique needs of our clients in the ever-changing global financial ecosystem.
DNBC Financial Group is your trusted provider in international money transfer
- Get 100% free 1-on-1 support
- 100% free account opening
- Seamless onboarding process
Or please contact DNBC
Email: [email protected]
Phone Number:
- +65 6572 8885 (Office)
- +1 604 227 7007 (Hotline Canada)
- +65 8442 3474 (WhatsApp)