Machine learning (ML) is shaping the financial industry with its ability to combine artificial intelligence (AI), big data, and advanced analytics.
It’s changing how financial institutions handle tasks like risk management and fraud detection, improving efficiency and accuracy.
This article explores the role of machine learning in finance, including how it uses data science blueprints and integrates AI and big data to redefine the finance sector.
The rise of machine learning in finance
ML involves using algorithms to identify patterns and insights from large datasets, enabling predictive modeling and decision-making.
The financial industry, known for its reliance on data, is an ideal environment for ML applications. A report by Grand View Research (2024) highlights the significant growth potential of ML in financial services. The global ML market in finance is expected to reach $41 billion by 2030, with a CAGR of 19.8% from 2023.
Over 70% of global financial institutions have already applied some form of machine learning to streamline operations.
How to apply artificial intelligence, machine learning and big data in finance? Here is the answer:
The applications of machine learning in finance
1. Fraud detection and prevention
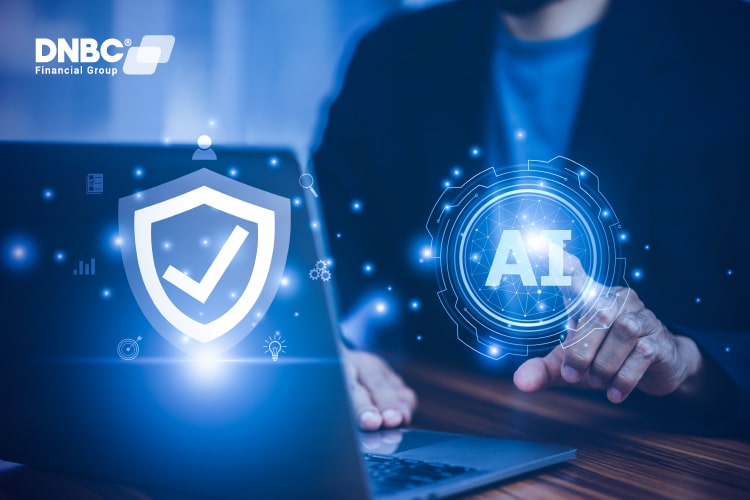
Fraud detection has always been a challenge for financial institutions. Machine learning provides a robust solution by analyzing transaction data in real time.
- How it works: ML algorithms detect anomalies in transaction patterns and flag potential fraudulent activities.
- Impact: According to the Federal Trade Commission (FTC), machine learning has reduced online payment fraud by 35% in 2023.
2. Risk management
Risk assessment is a core component of finance, and machine learning improves its accuracy dramatically.
- Credit scoring: ML models analyze customer credit histories, spending patterns, and other variables to determine creditworthiness.
- Market risk prediction: Algorithms process historical and real-time market data to identify potential risks and prevent losses.
3. Algorithmic trading
Algorithmic trading uses machine learning to execute trades based on predefined criteria.
- Advantages:
-
- Processes massive datasets quickly.
- Identifies market trends and opportunities faster than humans.
- Statistics: As of 2024, algorithmic trading accounts for 70% of equity market trades in the U.S., according to the Securities and Exchange Commission (SEC).
The integration of machine learning and data science blueprints for finance is guiding financial institutions toward more efficient processes. These blueprints offer frameworks for applying ML and data science techniques to address specific challenges.
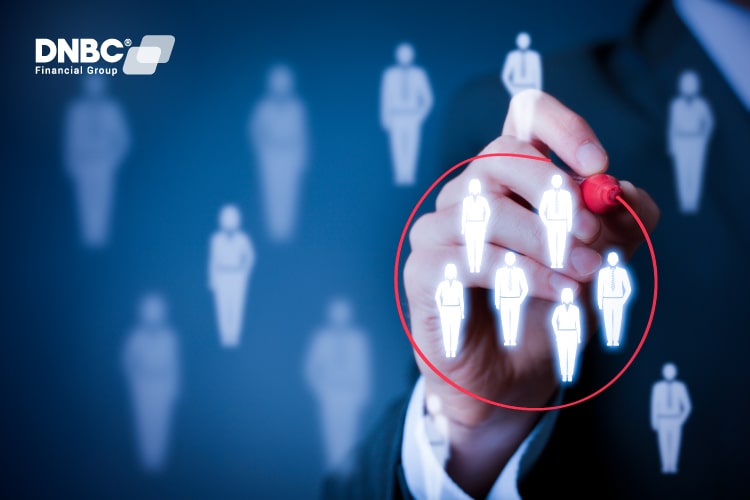
- Customer segmentation: Using data clustering techniques to segment customers based on behavior and preferences.
- Loan approval automation: Developing predictive models to automate loan approvals while minimizing default risks.
- Portfolio optimization: Enhancing investment strategies by balancing risk and return using ML algorithms.
Future trends in Machine Learning and Finance
The intersection of machine learning and finance continues to evolve. Here are some trends to watch in 2025 and beyond:
1. Decentralized finance (DeFi)
Machine learning is set to enhance DeFi platforms by improving risk assessment and fraud detection in blockchain-based financial systems.
2. Quantum computing
Quantum computing will accelerate machine learning processes, making predictions faster and more accurate.
3. Hyper-personalization
Financial institutions will leverage machine learning to offer hyper-personalized products and services based on customer behavior.
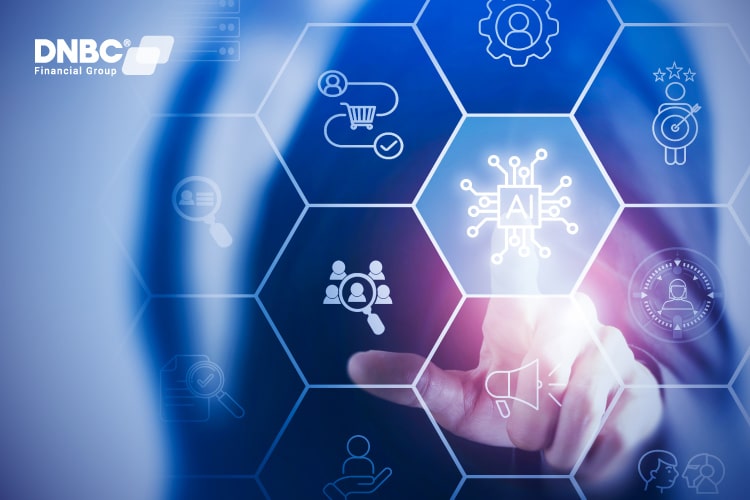
Machine learning and finance in DNBC Financial Group
DNBC Financial Group uses ML to transform its financial services and improve customer experiences. Nowadays, DNBC applies ML to make operations more efficient, manage risks, and provide personalized solutions.
Using advanced analytics and predictive modeling, DNBC identifies customer behavior patterns to create tailored financial products and services.
For example, real-time fraud detection systems powered by ML protect customers’ funds by quickly spotting and stopping suspicious activities. ML also improves DNBC’s global payment systems, ensuring faster and more accurate cross-border transactions, reducing delays and errors.
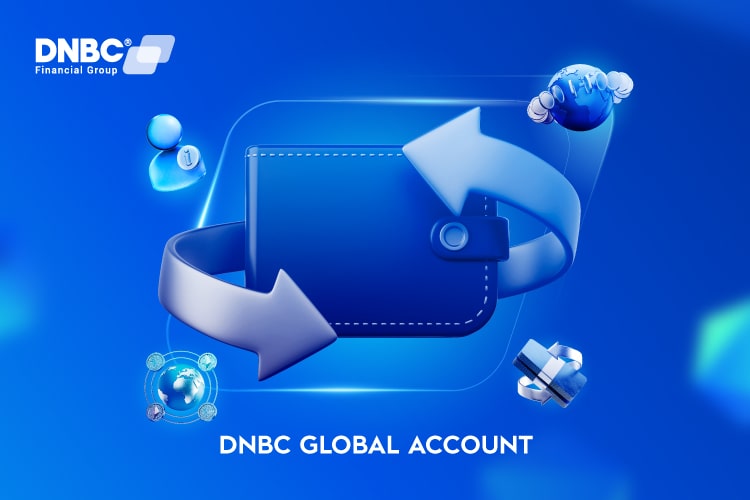
A key feature of DNBC’s services is dynamic currency exchange. ML algorithms analyze market trends to offer competitive exchange rates. This helps clients save money and build trust in their international financial operations.
DNBC also uses ML for portfolio optimization, helping clients achieve better financial results by analyzing market conditions and risks.
AI-powered chatbots and recommendation systems enhance customer support by providing fast and accurate assistance tailored to each client’s needs.
By adopting ML, DNBC Financial Group demonstrates how financial institutions can use technology to offer secure, efficient, and customer-focused solutions.
DNBC’s commitment to innovation makes it a leader in using machine learning in finance, helping clients succeed financially in a changing global market.
FAQs about Machine Learning and Finance
1. How is machine learning transforming the financial industry?
ML is revolutionizing finance by enhancing processes like fraud detection, risk management, algorithmic trading, and customer segmentation.
For example, ML algorithms analyze massive datasets to detect fraudulent transactions in real-time, reducing online payment fraud by 35% in 2023 (FTC report). It also streamlines operations and enables hyper-personalized financial solutions.
2. What are the benefits of using machine learning in financial institutions?
Machine learning improves efficiency, accuracy, and decision-making in finance. It helps financial institutions mitigate risks, optimize investment portfolios, and provide competitive currency exchange rates.
For instance, algorithmic trading, powered by ML, accounted for 70% of equity market trades in the U.S. in 2024, according to the SEC.
3. What role does DNBC Financial Group play in applying machine learning in finance?
DNBC Financial Group uses ML to enhance its financial services and customer experiences. It applies advanced analytics for fraud detection, risk management, and dynamic currency exchange.
DNBC also employs AI-powered chatbots and recommendation systems to provide personalized support, making it a leader in integrating machine learning into finance.
4. How does machine learning help with fraud detection and prevention?
Machine learning analyzes transaction patterns in real-time to identify anomalies and flag suspicious activities. This proactive approach has significantly reduced fraud cases in online payments. DNBC Financial Group uses ML-driven systems to protect customer funds and ensure secure financial transactions.
5. What future trends in machine learning and finance should businesses watch?
Key trends include the integration of quantum computing for faster predictions, the rise of decentralized finance (DeFi) platforms, and hyper-personalization of financial services. These advancements will enable financial institutions to offer more accurate, efficient, and customer-centric solutions. DNBC’s adoption of these trends positions it as a leader in the evolving financial landscape.
DNBC Financial Group is your trusted provider in international money transfer
- Get 100% free 1-on-1 support
- 100% free account opening
- Seamless onboarding process
Or please contact DNBC
Email: [email protected]
Phone Number:
- +65 6572 8885 (Office)
- +1 604 227 7007 (Hotline Canada)
- +65 8442 3474 (WhatsApp)