In recent years, machine learning applications in finance have emerged as a game-changer, reshaping the industry with unparalleled efficiency, accuracy, and innovation. From fraud detection to personalized customer experiences, machine learning (ML) enables financial institutions to harness vast data to make smarter, faster decisions. As financial markets evolve, integrating AI and machine learning in finance has become not just a competitive advantage but a necessity for staying ahead. This blog explores the top 10 machine learning applications that are revolutionizing the finance industry, offering a glimpse into how technology is redefining the future of finance.
Fraud Detection and Prevention
Fraud remains one of the most pressing challenges for financial institutions, costing the industry billions annually. Machine learning applications in finance are revolutionizing fraud detection by leveraging real-time data and advanced algorithms to identify suspicious activities. Unlike traditional rule-based systems, ML models can analyze vast datasets to uncover hidden patterns, anomalies, and behaviors indicative of fraud.
For example, deep learning, a subset of ML, excels in identifying subtle changes in transaction behavior that might escape human analysts or static systems. These deep learning use cases in finance allow organizations to detect and prevent fraudulent activities proactively before significant damage occurs.
A real-world application can be seen in how banks use ML to monitor credit card transactions. ML algorithms can instantly flag potential fraud by analyzing historical spending patterns and comparing them with real-time data. This not only protects customers but also enhances trust in financial systems. As AI and machine learning in finance continue to advance, fraud detection tools will become even more robust, ensuring a safer financial ecosystem for all stakeholders.
Credit Scoring and Risk Assessment
Accurate credit scoring and risk assessment are critical in ensuring financial stability and lending fairness. Traditional methods often rely on limited datasets and rigid criteria, which can overlook nuanced factors influencing a borrower’s creditworthiness. With the rise of machine learning applications in finance, these processes have been transformed, providing more comprehensive and precise evaluations.
To generate dynamic credit scores, machine learning models analyze diverse datasets, including transactional history, social behavior, and even alternative data like utility payments. These advanced models enable lenders to assess risk more accurately and extend credit to previously underserved groups. For instance, fintech companies leveraging AI and machine learning in finance can process applications faster and reduce bias in decision-making.
Deep learning further enhances risk assessment by identifying patterns and trends that traditional systems might miss. These deep learning use cases in finance allow for predictive analytics, helping financial institutions anticipate potential defaults and adjust their strategies proactively. By adopting ML, lenders minimize risks and build stronger relationships with their customers by offering tailored solutions.
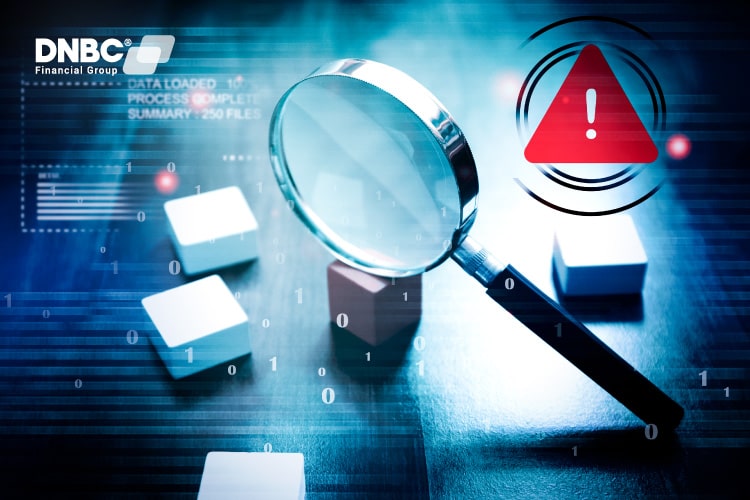
Algorithmic Trading
The world of trading has undergone a seismic shift with the advent of machine learning applications in finance, particularly in algorithmic trading. These sophisticated systems leverage ML algorithms to execute trades at lightning speed, using data-driven insights to predict market trends and optimize trading strategies.
Algorithmic trading powered by AI and machine learning in finance analyzes vast historical and real-time market data to identify profitable opportunities. For example, ML algorithms can detect price patterns and predict stock movements with accuracy that outperforms human traders. These tools are especially valuable in high-frequency trading, where split-second decisions can result in significant gains or losses.
Deep learning plays a pivotal role in enhancing algorithmic trading systems. Deep learning models provide traders with a more holistic view of market dynamics by processing complex datasets, such as social media sentiment and economic indicators. These deep learning use cases in finance help institutions stay competitive in volatile markets, delivering an edge in speed and precision.
As financial markets grow increasingly complex, algorithmic trading continues to showcase the transformative power of machine learning applications in finance, ensuring smarter, faster, and more profitable decision-making.
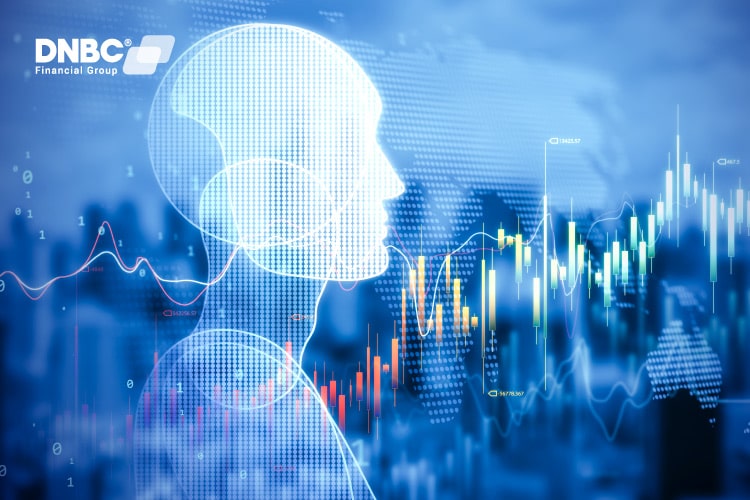
Personalized Customer Experiences
Customers expect personalized interactions in today’s digital-first world, even in the finance sector. Machine learning applications in finance are revolutionizing customer experiences by enabling financial institutions to deliver tailored solutions and proactive support. By analyzing customer data, ML algorithms can identify preferences, spending habits, and financial goals to offer personalized recommendations.
For instance, many banks and fintech companies now use AI and machine learning in finance to power chatbots and virtual assistants. These tools provide 24/7 support, answer queries, offer budgeting advice, or recommend investment opportunities based on individual profiles. Beyond support, ML also drives hyper-personalized marketing, ensuring customers receive relevant offers and promotions.
Deep learning further enhances personalization by using natural language processing (NLP) to understand customer sentiments and predict future needs. These deep learning use cases in finance enable institutions to foster stronger customer relationships, build trust, and increase satisfaction. By leveraging ML for personalized experiences, financial organizations improve retention and position themselves as customer-centric innovators.
Portfolio Management
Managing investment portfolios is another area where machine learning applications in finance are making a significant impact. Traditional portfolio management often requires manual analysis and relies heavily on historical data. ML, however, automates this process, providing real-time insights and adaptive strategies tailored to an investor’s risk tolerance and goals.
Robo-advisors, powered by AI and machine learning in finance, have become increasingly popular for managing portfolios. These systems use ML algorithms to analyze market trends, allocate assets, and rebalance portfolios automatically. The result is an efficient, cost-effective solution for retail and institutional investors.
Deep learning is key in portfolio optimization, as it processes vast datasets, including market indicators, geopolitical events, and social media sentiment. These deep learning use cases in finance help identify correlations and predict market movements, ensuring smarter investment decisions. For example, deep learning models can provide actionable insights into when to buy, hold, or sell assets.
By integrating ML into portfolio management, financial institutions empower investors with data-driven strategies, improving performance while reducing the need for manual intervention. This innovative approach highlights how technology continues to transform investment practices.
Compliance and Regulatory Technology (RegTech)
Navigating complex regulatory requirements is a significant challenge for financial institutions. Machine learning applications in finance transform compliance and regulatory processes, enabling organizations to meet stringent standards while reducing operational burdens. RegTech solutions, powered by AI and machine learning in finance, automate compliance tasks such as monitoring transactions, reporting suspicious activities, and ensuring adherence to evolving regulations.
ML algorithms can analyze vast datasets in real-time to identify anomalies and flag potential compliance issues, significantly reducing the risk of fines or reputational damage. For instance, advanced systems can sift through thousands of transactions to detect money laundering activities, which would take human analysts far longer to complete.
Deep learning is particularly valuable in this space, as it enhances natural language processing (NLP) for scanning legal documents, identifying relevant clauses, and ensuring compliance with specific regulations. These deep learning use cases in finance streamline audit preparation, allowing institutions to focus on growth rather than administrative tasks. By adopting ML-driven RegTech solutions, financial organizations can maintain regulatory integrity more efficiently and precisely.
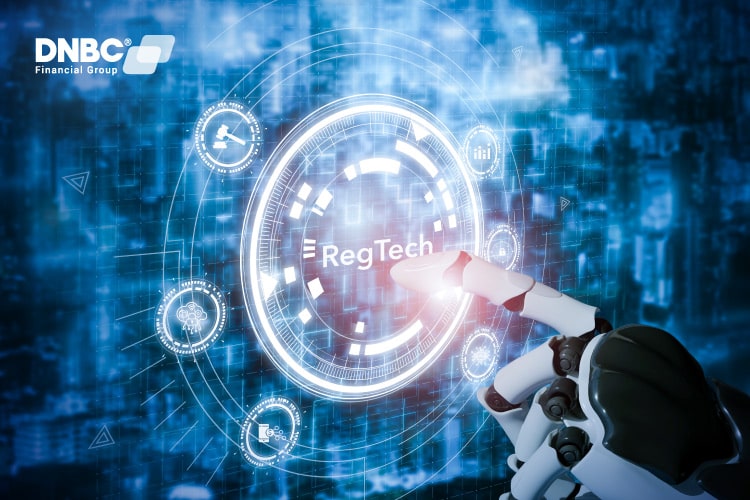
Predictive Analytics for Financial Markets
Predictive analytics revolutionizes analyzing financial markets, offering investors and institutions a competitive edge. Machine learning applications in finance leverage predictive models to analyze historical data, identify trends, and forecast future market behaviors. These insights empower traders and analysts to make data-driven decisions, minimizing risks and maximizing returns.
For example, ML algorithms can analyze stock prices, trading volumes, and macroeconomic indicators to predict market movements. Sentiment analysis, powered by AI and machine learning in finance, adds another layer of intelligence by evaluating news articles, social media posts, and other unstructured data to gauge market sentiment. This capability enables financial professionals to anticipate market shifts and adjust strategies proactively.
Deep learning is crucial in predictive analytics, as it processes complex, high-dimensional data to uncover intricate patterns and correlations. These deep learning use cases in finance help forecast asset prices, detect market anomalies, and optimize investment strategies. By utilizing predictive analytics, financial institutions can stay ahead of market trends, providing their clients with superior investment opportunities and strategic advice.
Risk Management
Effective risk management is essential for the stability of financial institutions. Machine learning applications in finance are revolutionizing this area by offering real-time insights and predictive capabilities that traditional methods cannot match. By analyzing vast datasets, ML models can identify potential risks, such as market volatility or operational failures, allowing organizations to take preemptive actions.
For example, banks use AI and machine learning in finance to assess credit, operational, and market risks. These models consider diverse factors, from historical data to current economic conditions, to generate more accurate risk profiles. Deep learning, a subset of ML, enhances this process by uncovering hidden patterns and relationships within complex datasets. These deep learning use cases in finance empower institutions to adapt to rapidly changing environments, ensuring better risk mitigation.
By integrating ML into risk management strategies, financial institutions can protect their assets and enhance decision-making processes, boosting confidence among stakeholders and regulators.
Loan Underwriting
The loan underwriting process, often considered time-consuming and subjective, has been significantly improved through machine learning applications in finance. ML algorithms evaluate a broader range of data, including traditional financial records, social behaviors, and alternative datasets, to provide a more comprehensive view of an applicant’s creditworthiness.
For instance, fintech companies leverage AI and machine learning in finance to automate loan approvals. These systems analyze data in real time, reducing the time it takes to approve loans and minimizing errors caused by manual evaluations. Deep learning further enhances this process by identifying subtle risk indicators that traditional models might miss, such as behavioral trends or spending patterns. These deep learning use cases in finance allow lenders to make informed decisions, offering fairer and faster access to credit.
By adopting ML-driven underwriting tools, financial institutions improve efficiency, reduce default rates, and expand their reach to underserved markets, ensuring a win-win for lenders and borrowers.
Document Processing and Automation
Managing and processing financial documents has historically been a labor-intensive task. Machine learning applications in finance have changed this landscape by automating document-related workflows improving efficiency and accuracy. From invoice processing to compliance documentation, ML-powered solutions transform how financial institutions handle large volumes of paperwork.
One of the key technologies used in this space is natural language processing (NLP), a subset of AI and machine learning in finance. NLP enables systems to extract relevant data from unstructured documents, such as contracts or invoices, and categorize it for further analysis. Deep learning models enhance this process by improving data extraction accuracy and recognizing context within complex documents. These deep learning use cases in finance allow institutions to save time, reduce errors, and lower operational costs.
For example, banks are using ML to automate the verification of customer documents during account opening or loan applications. This speeds up the process and ensures compliance with regulatory requirements. By implementing document automation tools, financial organizations can focus more on delivering value to their clients and less on administrative tasks.
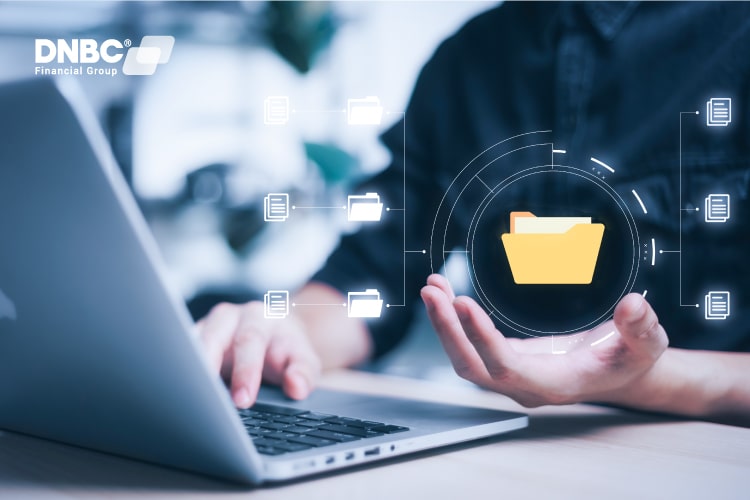
Conclusion
The transformative power of machine learning applications in finance is undeniable, reshaping the industry with innovative solutions for fraud detection, risk management, personalized customer experiences, and more. From enhancing decision-making processes to streamlining operations, AI and machine learning in finance offer unparalleled opportunities for financial institutions to stay competitive in an ever-evolving market. As these technologies advance, they pave the way for more innovative, efficient financial services.
At DNBC Financial Group, we are at the forefront of leveraging cutting-edge technology to simplify the complexities of payments and financial transactions. By integrating machine learning into our services, we provide our clients with secure, efficient, and personalized solutions suitable to their needs. Whether optimizing international money transfers, managing compliance, or enhancing user experiences, DNBC is committed to delivering innovation that empowers businesses and individuals.
Discover how DNBC Financial Group can help you confidently navigate the future of finance. Visit our website today to explore our comprehensive range of financial services. Together, let’s harness the power of technology to achieve your financial goals seamlessly.
DNBC Financial Group is your trusted provider in international money transfer
- Get 100% free 1-on-1 support
- 100% free account opening
- Seamless onboarding process
Or please contact DNBC
Email: [email protected]
Phone Number:
- +65 6572 8885 (Office)
- +1 604 227 7007 (Hotline Canada)
- +65 8442 3474 (WhatsApp)